MLL Research Symposium — Giannis Daras
Generative Models for Reconstruction, Art and Things in Between
A short introduction to Intermediate Layer Optimization
Generative Models for Reconstruction, Art and Things in Between
A short introduction to Intermediate Layer Optimization
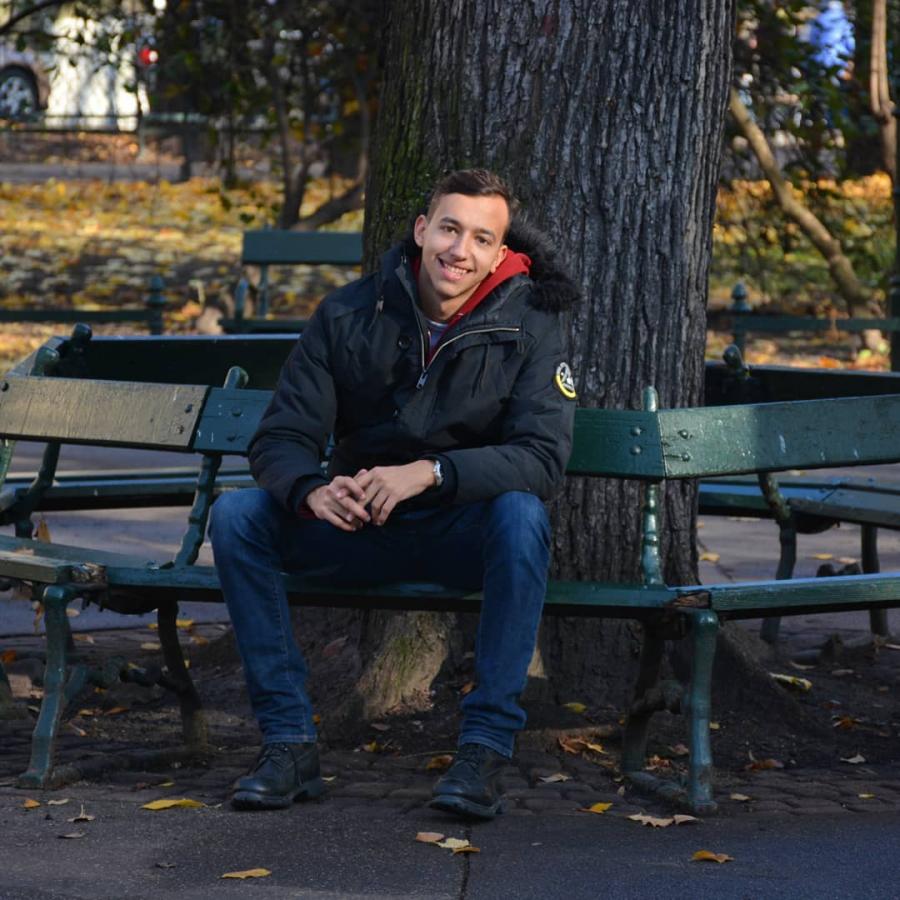
Abstract: Generative models are now capable of producing high fidelity images across many domains. The real question becomes what actual problems can we solve with these high-quality generators? We introduce a new framework, Intermediate Layer Optimization, that allows us to use pre-trained generative models to solve a variety of inverse problems, such as denoising, inpainting, style-transfer, text conditional image generation, etc. This talk includes empirical and theoretical results that show the flexibility, effectiveness and risks of this framework.
Speaker Bio: Giannis Daras is a second-year Ph.D. student at the Computer Science Department of UT Austin, supervised by Prof. Alexandros Dimakis. Giannis is also a Student Researcher at Google Research, working on score-based generative models. His research is on using deep generative models as priors to solve inverse problems. Giannis is interested in improving many aspects of generative modelling, including architecting better generative models, developing new optimization algorithms, understanding fundamental theoretical limits of reconstruction with deep priors and extending generative models to new modalities.