MLL Research Symposium — Sanjay Shakkottai
Representation Learning with Model-Agnostic Meta-Learning
Representation Learning with Model-Agnostic Meta-Learning
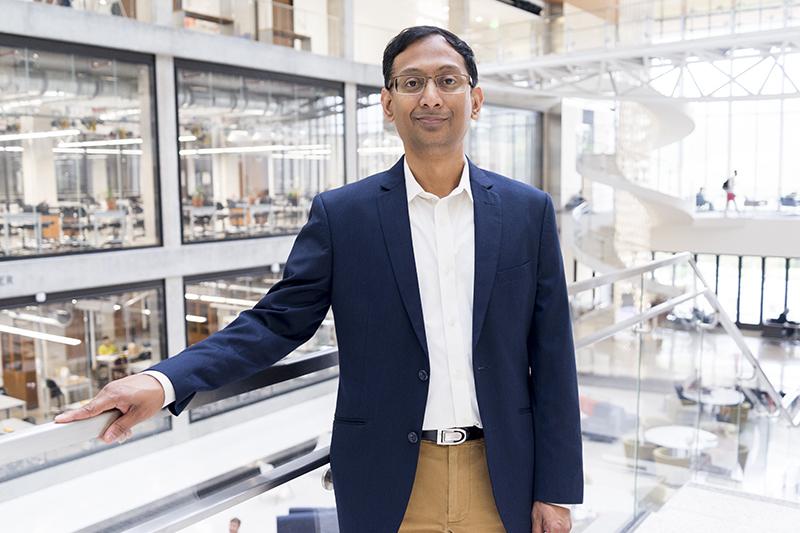
Abstract: Recent empirical evidence has driven conventional wisdom to believe that gradient-based meta-learning (GBML) methods perform well at few-shot learning because they learn an expressive data representation that is shared across tasks. However, the mechanics of GBML have remained largely mysterious from a theoretical perspective. In this talk, we show that GBML methods such as Model-Agnostic Meta-Learning (MAML) and its variants are capable of learning a common representation among a set of given tasks in the well-known multi-task linear representation learning setting. Moreover, our analysis illuminates that the driving force causing MAML to recover the underlying representation is that they adapt the final layer of their model, which harnesses the underlying task diversity to improve the representation in all directions of interest. To the best of our knowledge, these are the first results to show that MAML and its variants learn expressive representations and to rigorously explain why they do so. Based on joint work with Liam Collins, Aryan Mokhtari and Sewoong Oh.
Bio: Sanjay Shakkottai received his Ph.D. from the ECE Department at the University of Illinois at Urbana-Champaign in 2002. He is with The University of Texas at Austin, where he is a Professor in the Department of Electrical and Computer Engineering, and holds the Cockrell Family Chair in Engineering #15. He received the NSF CAREER award in 2004 and was elected as an IEEE Fellow in 2014. He was a co-recipient of the IEEE Communications Society William R. Bennett Prize in 2021. His research interests lie at the intersection of algorithms for resource allocation, statistical learning and networks, with applications to wireless communication networks and online platforms.