Ethics in AI Seminar - Facing an Adult Problem: New Data Sources for Fair Machine Learning
Join us Monday, April 26 at 1 pm CT! The Institute for Foundations of Machine Learning and Good Systems Challenge will welcome Moritz Hardt, Assistant Professor in Department of Electrical and Computer Science, University of California, Berkeley.
Join us Monday, April 26 at 1 pm CT! The Institute for Foundations of Machine Learning and Good Systems Challenge will welcome Moritz Hardt, Assistant Professor in Department of Electrical and Computer Science, University of California, Berkeley.
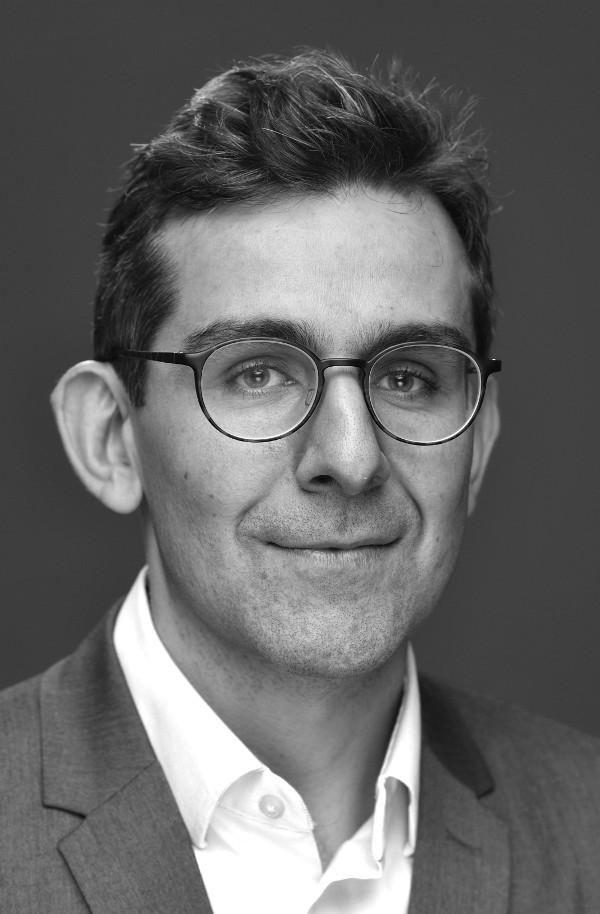
The Institute for Foundations of Machine Learning (IFML) and Good Systems are co-hosting a seminar series that examines use-inspired cases addressing the ethical foundations of AI/ML. We currently focus on fairness, whether it relates to bias, algorithmic justice, or another dimension of equity. Presentations hone in on the intersection of the technical and ethical challenges, and articulate how seemingly technical strategies might have broader societal impacts. Each presentation is followed by a dialogue among IFML and Good Systems affiliates, and guests. Join us Monday, April 26 at 1 pm CT! We will be welcome Moritz Hardt, Assistant Professor in Department of Electrical and Computer Science, University of California, Berkeley.
Monday, April 26, 2021
1 - 2 p.m. CT
Register at https://utexas.qualtrics.com/jfe/form/SV_6M4kqEhh2oYvdoW
Abstract
The research community investigating fairness and machine learning has recognized the importance of good data. When it comes to tabular data, however, most research papers on the topic continue to involve only a fairly limited collection of datasets, chief among them the UCI Adult data. Derived from 1994 US Census data, this small dataset has made an appearance in hundreds of research papers and served as the basis for the development and comparison of many algorithmic fairness interventions.
In this talk, Prof. Hardt will share some archaeology of the UCI Adult dataset, discuss its impact on the fairness community and highlight some of its limitations. He will then introduce the audience to a new collection of datasets derived from US Census data sources that vastly extend the existing data ecosystem for research on fair machine learning. These new datasets surface a range of empirical insights relevant to ongoing debates about statistical fairness criteria and algorithmic fairness interventions.
Speaker Bio
Moritz Hardt is an Assistant Professor in the Department of Electrical Engineering and Computer Sciences at the University of California, Berkeley. His research engages with the consequential interplay of algorithms, data, and society. Hardt obtained a PhD in Computer Science from Princeton University with a dissertation on privacy-preserving data analysis and fairness in classification. He then held research positions at IBM Research and Google.
Hardt co-founded the Workshop on Fairness, Accountability, and Transparency in Machine Learning. He is a co-author of "Fairness and Machine Learning: Limitations and Opportunities" and "Patterns, Predictions, and Actions: A Story about Machine Learning". He has received an NSF CAREER award, a Sloan fellowship, and best paper awards at ICML 2018 and ICLR 2017.
Contact us: IFML@austin.utexas.edu