Recap: Machine Learning Lab Research Symposium 2024
In April, we celebrated the opening of the new Texas Center for Generative AI with a two-day research symposium that convened Generative AI experts from academia and industry. Watch the recordings!
In April, we celebrated the opening of the new Texas Center for Generative AI with a two-day research symposium that convened Generative AI experts from academia and industry. Watch the recordings!
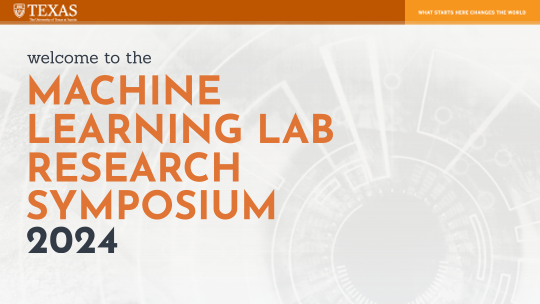
In January, UT Austin announced the launch of its new Texas Center for Generative AI, led by IFML co-director Alex Dimakis. With 600 NVIDIA H100s GPUs, the Center is home to one of the largest GPU computing clusters in academia. To celebrate the new center, the Machine Learning Lab expanded its annual research symposium to convene faculty, students and industry experts in Generative AI. Keynotes from Sébastien Bubeck (VP of GenAl at Microsoft Research) and Luke Zettlemoyer (Professor at the University of Washington and Research Scientist at Meta) kicked off two days of presentations and discussions about large (and small) language models, open source tools, protein engineering, and the future of AI across industries.
Day 1:
Keynote: Sébastien Bubeck, VP of GenAl at Microsoft Research
Small Language Models: Large language models (LLMs) have taken the field of AI by storm. But how large do they really need to be? I'll discuss the phi series of models from Microsoft, which exhibit many of the striking emergent properties of LLMs despite having merely a few billion parameters.
Dan Roth, VP/Distinguished Scientist, AWS AI & The Eduardo D. Glandt Distinguished Professor, The University of Pennsylvania
Reasoning Myths about Language Models: What is Next?: Performance of language models and higher-order reasoning. On the road to AGI, have we solved it all?
Panel Discussion on Generative AI: A comprehensive discussion on the current state and future of generative AI in academia and industry
Sujay Sanghavi (Moderator)
Scott Aaronson, Professor of Computer Science, UT Austin
Greg Durrett, Associate Professor of Computer Science, UT Austin
Amir Husain, Founder, SparkCognition
Dayran Dehghanpisheh: President & Co-Founder, Protect AI
Securing LLMs: OpenAI's GPT-4 and other LLMs are revolutionizing AI. Their adoption spans various sectors, including customer service, healthcare, and content creation, driving the market's growth from USD 11.3 billion in 2023 to an expected USD 51.8 billion by 2028, according to multiple industry analysts. This growth, fueled by the demand for applications like chatbots and virtual assistants, positions LLMs as key tools for businesses seeking to leverage textual data for competitive advantage. However, security and misuse concerns are limiting wider adoption among major companies.
Sanjay Shakkottai, Professor, ECE, UT Austin
On Solving Inverse Problems Using Latent Diffusion-based Generative Models: Diffusion models have emerged as a powerful new approach to generative modeling. In this talk, we present the first framework that uses pre-trained latent diffusion models to solve linear inverse problems such as image denoising, inpainting, and super-resolution. Previously proposed algorithms (such as DPS and DDRM) only apply to pixel-space diffusion models. We theoretically analyze our algorithm showing provable sample recovery in a linear model setting. The algorithmic insight obtained from our analysis extends to more general settings often considered in practice. Experimentally, we outperform previously proposed posterior sampling algorithms in a wide variety of problems including random inpainting, block inpainting, denoising, deblurring, destriping, and super-resolution. Next, we present an efficient second-order approximation using Tweedie's formula to mitigate the bias incurred in the widely used first-order samplers. With this method, we devise a surrogate loss function to refine the reverse process at every diffusion step to address inverse problems and perform high-fidelity text-guided image editing. Based on joint work with Litu Rout, Negin Raoof, Giannis Daras, Constantine Caramanis, Alex Dimakis, Yujia Chen, Abhishek Kumar, and Wen-Sheng Chu.
Day 2:
Keynote: Luke Zettlemoyer, Professor at The University of Pennsylvania and Research Scientist at Meta
Branch-Train-Merge: Embarrassingly Parallel Training of Expert Language Models--Existing language model (LM) training regimes entangle compute, data, and parameters, requiring expensive synchronous communication with massive supercomputers. This talk introduces a new algorithm called Branch-Train-Merge (BTM) that asynchronously trains LMs that are fundamentally modular. In BTM, components (or experts) of the LM are specialized to distinct domains in the training corpus, and experts are conditionally updated based on the domain of the incoming document. We show how BTM enables LMs that are rapidly customizable (with the ability to mix, add, or remove experts after training), embarrassingly parallel (requiring no communication between experts), and sparse (needing only a few experts active at a time for inference). Key to our proposal is exploring what constitutes the domains to which experts specialize, as well as reflecting on the data sources used to train LMs. Our new techniques chart a path towards collaborative and iterative LM development, where anyone can contribute and maintain experts at modest computational cost.
Panel: Navigating Intersection: AI’s Role in Shaping the Secure Open Source Software Ecosystem--Join us as we navigate these intersections, exploring the relationship between AI, security, and open-source software (OSS) could drive innovation, enhancing security, and fostering a more robust open-source community. This talk will emphasize the interconnectedness of AI, security, and OSS, and the transformative potential of these intersections.
Harry Toor (Moderator) Chief of Staff, The Linux Foundation
Zaib Husain, Founding Partner, Global Venture Bridge
Alex Dimakis, Professor, The University of Texas at Austin
Danny Diaz, IFML Post-doctoral Researcher, UT Austin
Learning how Evolution Engineers Proteins: Protein engineering enables scientists to address medical, chemical, and environmental issues by converting natural proteins into biotechnologies. Currently, this process is more stochastic than deterministic and often fails to generate proteins sufficient for commercialization. However, nature has been evolving proteins for nearly 4 billion years with tremendous success. Here, we present novel machine learning techniques designed to capture how nature evolves proteins and demonstrate their ability to accelerate the engineering of protein-based biotechnologies.
Stella Offner, Associate Professor of Astronomy, UT Austin
Advancing New Frontiers in Astronomy Data Analysis and Discovery with AI: Recent advances in large language models (e.g., ChatGPT) will transform how astronomers interact with data and how astronomy discoveries are made. Prof. Offner will describe studies that apply computer vision and generative AI to tackle fundamental star formation questions: How does stellar feedback impact molecular clouds? What is the past and future evolution of star-forming regions?