AI Health Invited Talk Series: Oct 3
October 3, 2024 at 12:00PM
Tianlong Chen, Assistant Professor, The University of North Carolina at Chapel Hill
Title: Flexible Modality Learning: Modeling Arbitrary Modality Combination via the Mixture-of-Experts Framework
Join via Zoom: https://utexas.zoom.us/j/5128555388
RSVP: https://utexas.qualtrics.com/jfe/form/SV_7PAD9kKLANVSYOq
October 3, 2024 at 12:00PM
Tianlong Chen, Assistant Professor, The University of North Carolina at Chapel Hill
Title: Flexible Modality Learning: Modeling Arbitrary Modality Combination via the Mixture-of-Experts Framework
Join via Zoom: https://utexas.zoom.us/j/5128555388
RSVP: https://utexas.qualtrics.com/jfe/form/SV_7PAD9kKLANVSYOq
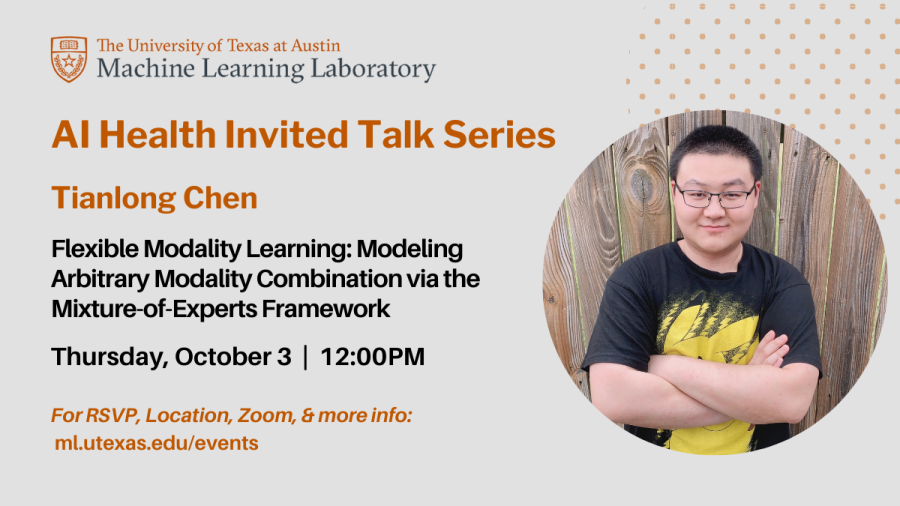
__________
The Center for Generative AI is excited to launch the Fall 2024 AI Health Invited Talk Series. The seminars will take place on Thursdays at 12pm at Dell Medical School and will consist of a 30 minute hybrid Zoom talk followed by a 30 minute in-person lunch discussion (lunch will be provided).
The goal of the lunch discussion is to connect computer scientists and engineers with clinicians to tackle actionable AI/Health projects. We ask that you kindly fill out the RSVP form if you are interested in attending the in-person lunch discussion. While the talk is open to everyone, due to limited space the in-person lunch will be provided to those who RSVP under a first-come, first-serve basis until we reach capacity.
RSVP here to attend in person.
Zoom link to join virtually: https://utexas.zoom.us/j/5128555388
__________
Fall 2024 Speakers & Locations:
Sept 19: Carl Yang, Emory | HDB 1.202
Oct 3: Tianlong Chen, UNC | HDB 1.204
Oct 17: Akshay Chaudhari, Stanford | HLB 1.111 Auditorium
Oct 31: Grett Durrett, UT | HDB 1.202
Nov 14: Ziyue XU, Nvidia Health | HDB 1.202
__________
This Week's Speaker: Tianlong Chen, Assistant Professor, The University of North Carolina at Chapel Hill
Title: Flexible Modality Learning: Modeling Arbitrary Modality Combination via the Mixture-of-Experts Framework
Abstract: While multimodal approaches are essential in understanding biological and disease behaviors, existing works primarily focus on single modalities such as imaging, genomics, or clinical data. Few recent studies attempt to integrate multiple modalities, and the lack of robust methods to handle missing modalities often limits the usability of such integrative approaches, forcing reliance on samples where the target modalities are available. Addressing this gap, we propose a novel multimodal learning framework, Flex-MoE (Flexible Mixture-of-Experts), which is flexible in incorporating diverse modalities in healthcare research while being resilient to missing modality scenarios through an advanced Sparse MoE design. This talk will illustrate the core idea of Flex-MoE, and demonstrate its effectiveness in handling diverse missing modality scenarios.
Speaker Bio: Dr. Tianlong Chen is an assistant professor in the Department of Computer Science at the University of North Carolina at Chapel Hill. He received his Ph.D. degree from ECE at UT Austin in 2023. His research focuses on efficient and reliable machine learning, graph learning, learning-augmented optimization, and AI4Science. His paper has received the Best Paper Award from the inaugural Learning on Graphs (LoG) Conference 2022. His work has been cited more than 8,800 times with an h-index of 44. He has served as Area Chair for diverse top conferences like ICLR’25, EMNLP’24, ICIP, CPAL, etc.