AI Health Invited Talk Series: 10/31
October 31, 2024 at 12:00PM
Greg Durrett, Associate Professor, The University of Texas at Austin
Title: Specializing LLMs for Factuality and Soft Reasoning
Join via Zoom: https://utexas.zoom.us/j/5128555388
RSVP: https://utexas.qualtrics.com/jfe/form/SV_7PAD9kKLANVSYOq
October 31, 2024 at 12:00PM
Greg Durrett, Associate Professor, The University of Texas at Austin
Title: Specializing LLMs for Factuality and Soft Reasoning
Join via Zoom: https://utexas.zoom.us/j/5128555388
RSVP: https://utexas.qualtrics.com/jfe/form/SV_7PAD9kKLANVSYOq
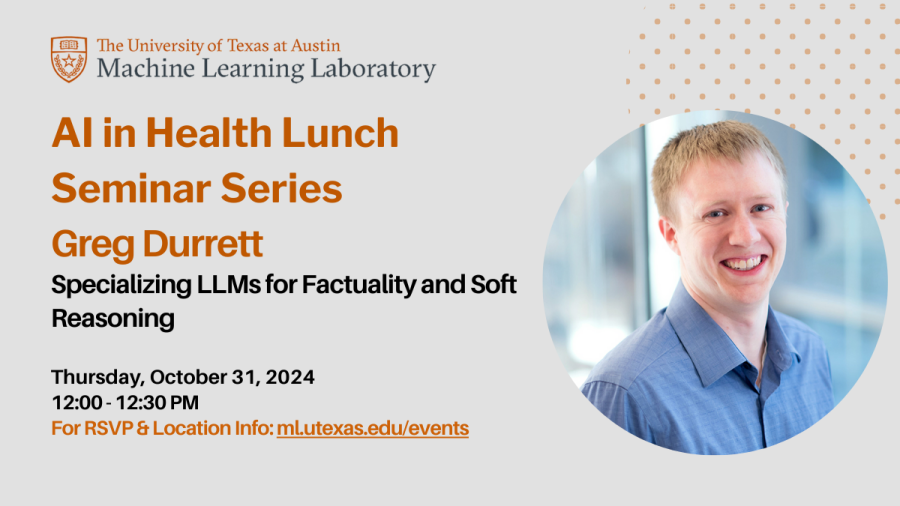
The Center for Generative AI is excited to launch the Fall 2024 AI Health Invited Talk Series. The seminars will take place on Thursdays at 12pm at Dell Medical School and will consist of a 30 minute hybrid Zoom talk followed by a 30 minute in-person lunch discussion (lunch will be provided).
The goal of the lunch discussion is to connect computer scientists and engineers with clinicians to tackle actionable AI/Health projects. We ask that you kindly fill out the RSVP form if you are interested in attending the in-person lunch discussion. While the talk is open to everyone, due to limited space the in-person lunch will be provided to those who RSVP under a first-come, first-serve basis until we reach capacity.
RSVP here to attend in person.
Zoom link to join virtually: https://utexas.zoom.us/j/5128555388
__________
Fall 2024 Speakers & Locations:
Sept 19: Carl Yang, Emory | HDB 1.202
Oct 3: Tianlong Chen, UNC | HDB 1.204
Oct 17: Akshay Chaudhari, Stanford | HLB 1.111 Auditorium
Oct 31: Grett Durrett, UT | HDB 1.202
Nov 14: Ziyue XU, Nvidia Health | HDB 1.202
__________
This Week's Speaker: Greg Durrett, Associate Professor, The University of Texas at Austin
Title: Specializing LLMs for Factuality and Soft Reasoning
Abstract: Proponents of LLM scaling assert that training a giant model on as much data as possible can eventually solve most language tasks, perhaps even leading to AGI. However, frontier LLMs still fall short on complex problems in long-tail domains like healthcare. Errors occur somewhere in the process of encoding the necessary knowledge, surfacing it for a specific prompt, and synthesizing it with other input data. In this talk, I will argue that specialization is the right approach to improve LLMs here; that is, modifying them through training or other means to improve their factuality and reasoning capabilities. First, I will show that specialization is necessary: inference-only approaches like chain-of-thought prompting are not sufficient. Second, I will present our fact-checking system MiniCheck, which is fine-tuned on specialized data to detect factual errors in LLM responses, leading to a better detector than frontier models like GPT-4. Finally, I will conclude by discussing some potential paths forward to create better systems for LLM reasoning for domains like healthcare.
Speaker Bio:Greg Durrett is an associate professor of Computer Science at UT Austin. He received his BS in Computer Science and Mathematics from MIT and his PhD in Computer Science from UC Berkeley, where he was advised by Dan Klein. His research is broadly in the areas of natural language processing and machine learning. Currently, his group's focus is on techniques for reasoning about knowledge in text, verifying factuality of LLM generations, and building systems using LLMs as primitives. He is a 2023 Sloan Research Fellow and a recipient of a 2022 NSF CAREER award. He has co-organized the Workshop on Natural Language Reasoning and Structured Explanations at ACL 2023 and ACL 2024, as well as workshops on low-resource NLP and NLP for programming. He has served in numerous roles for *CL conferences, including as a member of the NAACL Board since 2024.