IFML Seminar: Variational Autoencoding Neural Operators
Join us Friday, March 31 at 12:15 pm CT! The Institute for Foundations of Machine Learning will welcome Paris Perdikaris, Assistant Professor, University of Pennsylvania.
Join us Friday, March 31 at 12:15 pm CT! The Institute for Foundations of Machine Learning will welcome Paris Perdikaris, Assistant Professor, University of Pennsylvania.
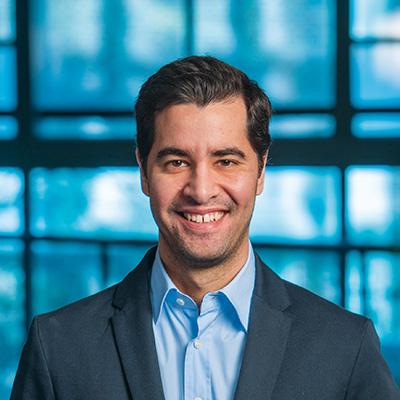
12:15-1:00 pm CT
Register at https://utexas.qualtrics.com/jfe/form/SV_0Vq6vC6KlergHoa
Abstract:
Unsupervised learning with functional data is an emerging paradigm of machine learning research with applications to computer vision, climate modeling and physical systems. A natural way of modeling functional data is by learning operators between infinite dimensional spaces, leading to discretization invariant representations that scale independently of the sample grid resolution. Here we present Variational Autoencoding Neural Operators (VANO), a general strategy for making a large class of operator learning architectures act as variational autoencoders. For this purpose, we provide a novel rigorous mathematical formulation of the variational objective in function spaces for training. VANO first maps an input function to a distribution over a latent space using a parametric encoder and then decodes a sample from the latent distribution to reconstruct the input, as in classic variational autoencoders. We test VANO with different model set-ups and architecture choices for a variety of benchmarks. We start from a simple Gaussian random field where we can analytically track what the model learns and progressively transition to more challenging benchmarks including modeling phase separation in Cahn-Hilliard systems and real world satellite data for measuring Earth surface deformation. We also show that conditional formulations of VANO can be used to tackle inverse problems where not all functional outputs of a system are available for observation.
*Joint work with: Jacob H. Seidman, Georgios Kissas and George J. Pappas
Bio:
Paris Perdikaris is an Assistant Professor in the Department of Mechanical Engineering and Applied Mechanics at the University of Pennsylvania. He received his PhD in Applied Mathematics at Brown University in 2015, and, prior to joining Penn in 2018, he was a postdoctoral researcher at the department of Mechanical Engineering at the Massachusetts Institute of Technology. His current research interests include physics-informed machine learning, uncertainty quantification, and engineering design optimization. His work and service has received several distinctions including the DOE Early Career Award (2018), the AFOSR Young Investigator Award (2019), the Ford Motor Company Award for Faculty Advising (2020), the SIAG/CSE Early Career Prize (2021), and the Scialog Fellowship (2021).